Case studies
Take a look at our projects
Develop a product that makes a difference with people who can do the impossible
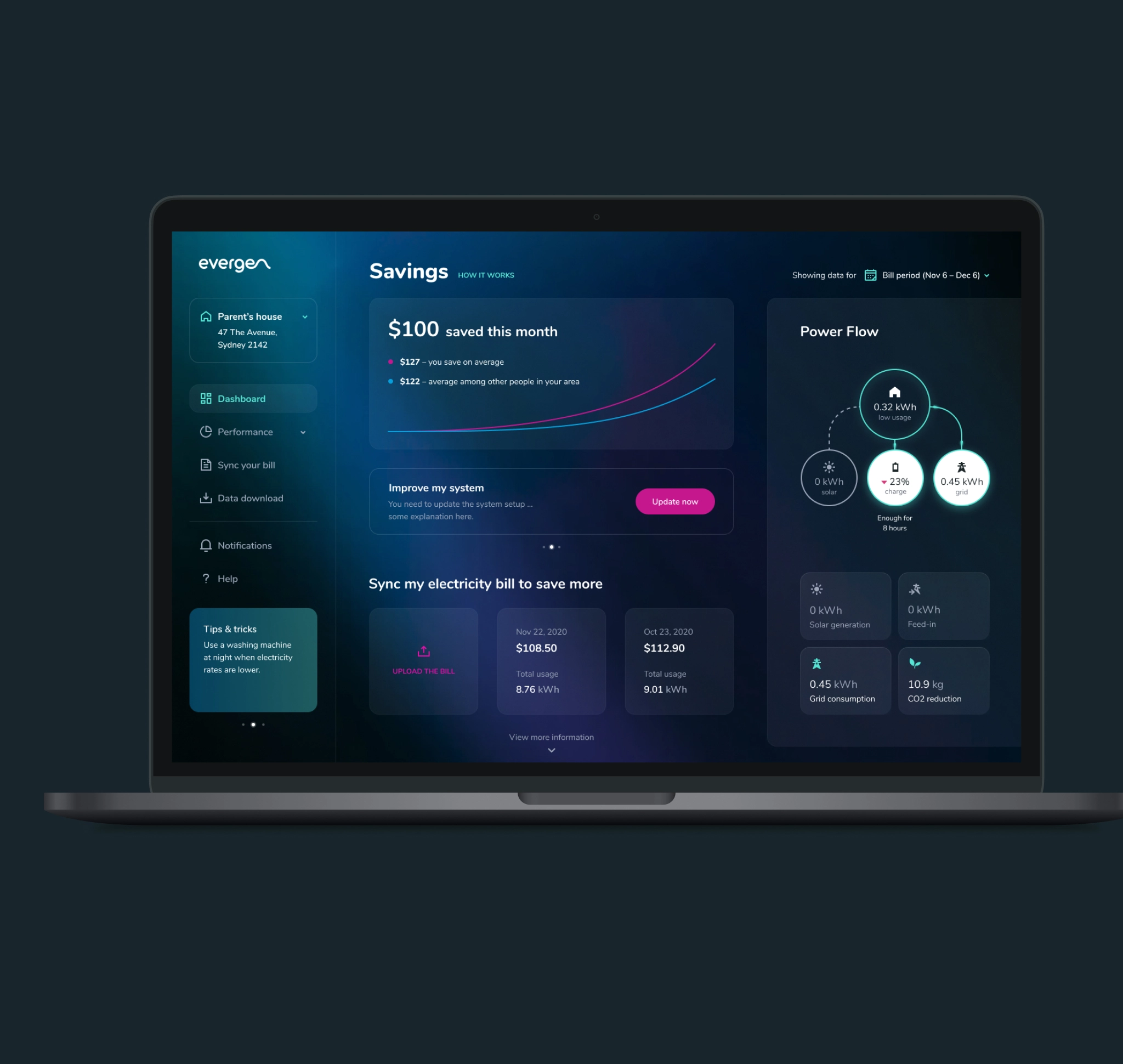
Evergen
Large-scale digital transformation for the energy sector
5X accelerated system installations
Automation of business processes
Improved visibility into business processes
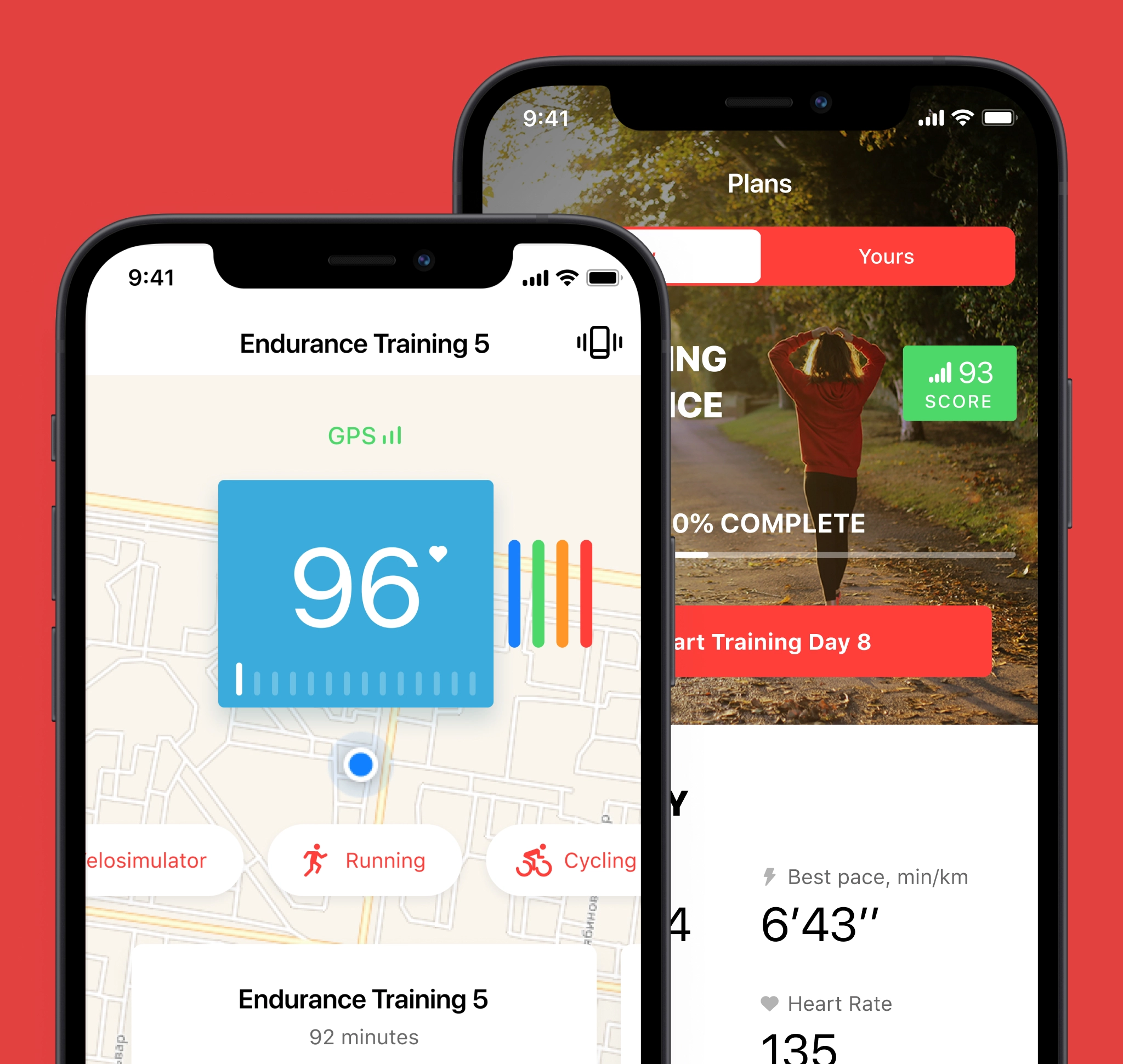
Smartrun
A personal running coach for improving heart health
Constant HR monitoring
Real-time GPS tracking
HealthKit integration
RealTime offline personalised AI feedback
Big data training analyses and anomaly detection
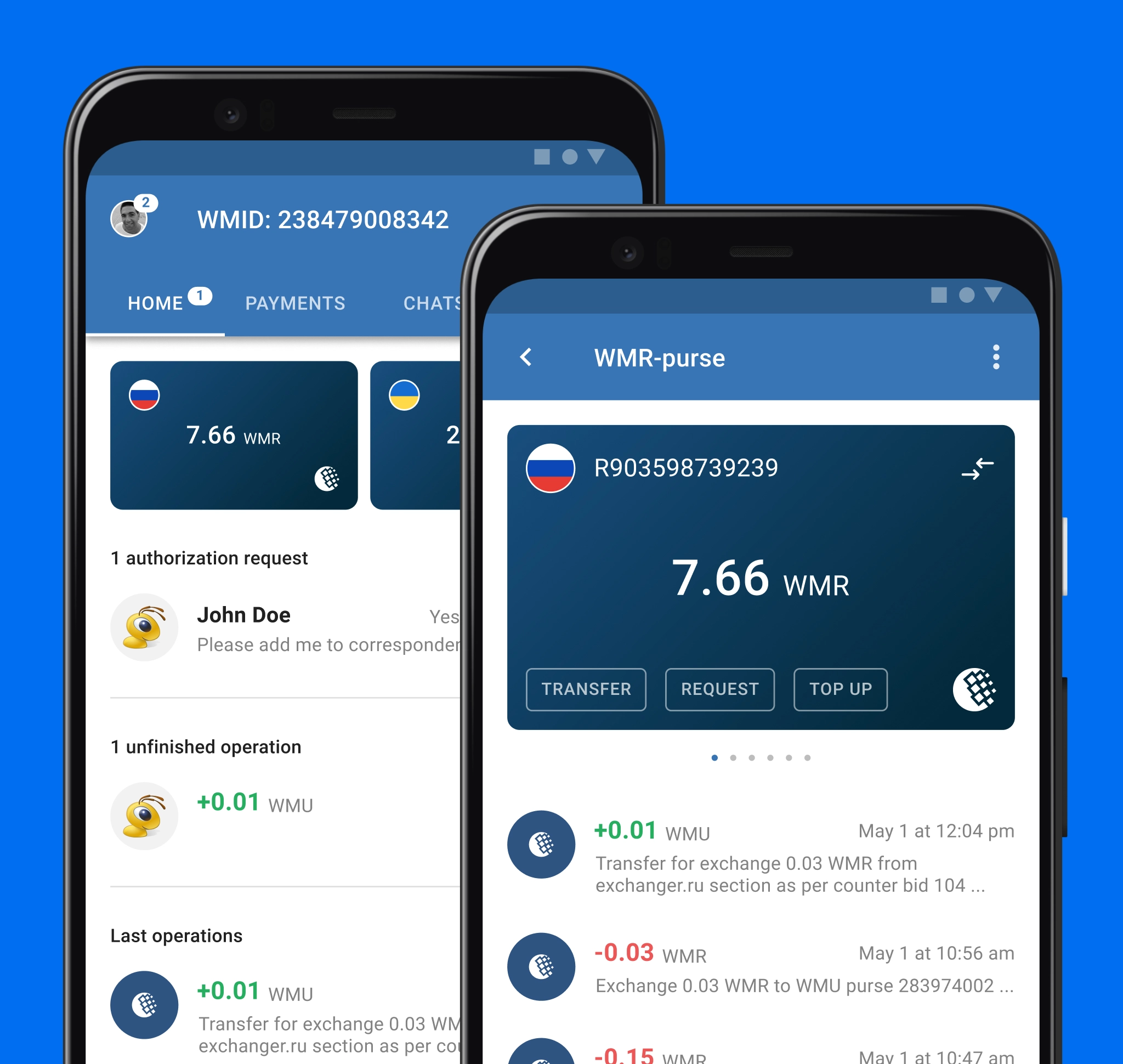
Webmoney
Developing a mobile app with bank-grade security for a global payment system
Protected against malicious attacks
Consistent UX across the web, Android, Windows, and even Symbian
Used by millions of people

Domino's Pizza Australia
Improving user experience of Domino's Pizza mobile app for the Australian market to increase user's satisfaction
Full-cycle Design Process
CSAT, SUS, NPS, TOT metrics
Moderated Usability Testing